AI at the IoT Edge Is Disrupting the Industrial Market




Artificial intelligence (AI) at the edge of the network is a cornerstone that will influence the future direction of the technology industry. If AI is an engine of change, then semiconductors are the oil driving the new age that is being defined by machine learning (ML), neural networks, 5G connectivity, and the advent of blockchain, digital twins, and the metaverse.
Despite recent disruptions to the chip industry due to supply chain and more recently, macroeconomic factors, the confluence of AI – and the Internet of Things (IoT) known as AIoT– is poised to shift the world from cloud-centric intelligence to a more distributed intelligence architecture.
It is expected that a staggering 73.1 zettabytes of data are expected to be generated by IoT devices, in 2025 according to IDC Research. As a result, endpoint data will increase at a CAGR of 85% from 2017 to 2025, driving intelligence from the cloud to the endpoint to run AI/ML workloads within tiny machines (TinyML). Some of the applications that are seeing the most disruption include the development of "voice as a user interface" to improve human-to-machine communication, as well as environmental sensing and predictive analytics and maintenance. Major growth segments include wearables, smart homes, smart cities, and intelligent industrial automation.
What are the benefits of embedding intelligence at the endpoint? Many industrial IoT applications operate within environments constrained by memory capacity, limited coMPUting, battery power, and sub-optimal connectivity. Moreover, these applications often require real-time responses that may be mission and system critical. Expecting such devices and applications to operate in a cloud-centric intelligence architecture just does not work.
This is where the power of embedding intelligence at the endpoint is evolving from standard industrial IoT implementations to what RENESAS is calling AIoT for industrial applications.
Transforming data at the source of collection minimizes latency and enables optimized processing for time-critical applications. Because data is not processed and transported over the network, the security concerns related to the transfer and flow of data, are greatly minimized. Another advantage is that data handling can be linked with root-of-trust at the endpoint, making the implementation impervious to attacks. Since data processing is handled at or very near the source, Renesas can fully leverage data gravity and reduce the power consumption associated with turning on radios or moving data through the network.
Renesas' commitment to customers is to lead the industry in endpoint computing technology with the broadest range of MCUs and MPUs. Already this has enabled designers to leverage Renesas' rich ecosystem of IoT and AI/ML building blocks by tapping into a technology ecosystem that features more than 300 building blocks of commercial-grade software provided by Renesas's trusted partners.
Renesas growing AIoT portfolio also explains Renesas' recent acquisition of Reality AI, a new platform powering edge and endpoint AI in industrial IoT applications using Renesas processors. Reality AI automatically searches a wide range of signal-processing transforms and generates custom machine learning models while retaining traceability in its approach and offering valuable hardware design analytics. The models run on nearly every MCU and MPU core available from Renesas – with new ones added constantly.
This puts an incredibly powerful tool into the hands of designers to help them solve their most difficult problems because the model development is specifically for non-visual sensing use cases and is based on advanced signal processing math and edge deployment. This enables advanced analytics capable of supporting full hardware design and complete frameworks, including data collection, instrumentation, firmware, and ML workflows. Other solutions simply generate algorithms and models that often account for only 5% of typical project costs, while ignoring the other 95% of development expenses.
Renesas' comprehensive approach to AIoT design allows developers to reduce unscheduled equipment downtime, improve production efficiencies and perform sophisticated quality assurance tasks that are costly or difficult to replicate in a current testing environment.
In a real-world use case tested under 51 different environmental and load conditions in a three-ton residential HVAC system, Reality AI was able to achieve a greater than 95% accuracy when detecting and distinguishing single fault conditions. The test also detected indoor and outdoor air-flow blockage and charge faults as small as 5% from OEM specifications in both heating and cooling modes.
The convergence of AI and IoT for industrial applications is a megatrend with significant potential. The acquisition of Reality AI unlocks the potential of combining advanced signal processing with AI at the edge and is supported by Renesas' extensive hardware, software, tools, and ecosystem to provide all the building blocks you need to unleash your creativity.
- |
- +1 赞 0
- 收藏
- 评论 0
本文由Vicky转载自Renesas Blogs,原文标题为:Executive Blog: AI at the IoT Edge Is Disrupting the Industrial Market,本站所有转载文章系出于传递更多信息之目的,且明确注明来源,不希望被转载的媒体或个人可与我们联系,我们将立即进行删除处理。
相关研发服务和供应服务
相关推荐
端点人工智能给嵌入式视觉应用带来革命性创新
现实世界中有无数AI视觉应用的使用场景,Renesas可以提供全面的MCU和MPU解决方案,包括必要的软件和工具,以实现快速开发。
行业资讯 发布时间 : 2022-07-27
世强目前有代理riscv的mcu吗?
世强代理的RISC-V内核MCU厂家越来越多,还在不断增长。 国外厂家有瑞萨 【产品】瑞萨推出全新RISC-V MCU R9A02G020,优化先进电机控制系统设计和降低用户开发成本 国内厂家介绍如下: 广芯微内置32位RISC-V内核的PD SoC芯片UM3506,最高主频33MHz 全球首家全栈自研RISC-V内核的通讯接口芯片/全栈MCU供应商沁恒(WCH) RISC-V内核低功耗32位MCU,中移芯昇授权世强先进全线代理 芯昇科技携多款芯片产品亮相ICDIA,助力RISC-V生态发展 先楫半导体提供多系列通用MCU,以开源的RISC-V架构为核心,综合性能达世界领先水平 航顺芯片首次进入胡润全球独角兽榜,已量产中国第一颗M3+RISC-V多核MCU 中微半导体积极推动RISC-V架构安全化、智能化,共筑国产安全芯片生态 博流业界第一款基于RISC-V CPU的WI-FI+BLE双模SoC芯片,打造智慧家居AIoT芯片平台 璇玑CLE系列是核芯互联基于32位RISC-V内核推出的通用嵌入式MCU处理器 方寸微电子加入RISC-V产业联盟,推动安全芯片国产化,打破国外芯片技术垄断
技术问答 发布时间 : 2019-12-09
Silicon Labs Extends Award-Winning xG22 Platform to Deliver Optimized 32-Bit MCU for IoT Edge Applications
March 3, 2021 – Silicon Labs announced the launch of the EFM32PG22 (PG22) 32-bit microcontroller unit (MCU), a low-cost, high-performance solution with an industry-leading combination of energy efficiency, performance and security. With easy-to-use, cost-efficient analog capabilities, the PG22 MCU is ideally suited for rapid development of consumer and industrial applications with demanding size constraints and low-power operation requirements.“
新产品 发布时间 : 2021-03-23
Silicon Labs New 32-bit PG23 MCUs Bring Ultra-Low Power and High Performance to Embedded IoT Applications
Silicon Labs’PG23 is a 32-bit microcontroller (MCU) that offers best-in-class security, extremely low-power operation, and software compatibility with the company’s broad portfolio of wireless SoCs. This combination makes it a good fit for industrial IoT applications.
新产品 发布时间 : 2022-11-11
Renesas(瑞萨)电机控制微控制器(MCU)选型指南
目录- 电机MCU路线图 电机驱动推荐微控制器 16位RL78电机控制MCU RX-T电机控制MCU RA-T电机控制MCU RA-T系列主要特性 RISC-V电机专用MCU 电机控制解决方案
型号- RX200,RX6XT,RX23T,RX71M,RX2XT,RA6T3 GROUP,RISC-V-MC,RA-T,RA-T系列,RX600,RX6XX,RX63T,RX66T,RA4T1,RA6T2,RX66N,RA6T1,RX26T,RA8T2,RA6T3,RA8T1,RX62G,RA4T1 GROUP,RX-T,RA,MCK-XXXXX,RL78/G14,RA6T1 GROUP,RL78,RX74T,RX62T,RX65X,RX13T,RX7XT,RX,RX100,RISC-V,RL78/G1F,RL78/G1G,RX1XT,RL78/G24,RX7XX,RA6T2 GROUP,RX24T,RX72M,RX24U,RX72N,RA2,RA4,RXV2,RXV3,RA6,RXV1,RA8,RA-T 系列,RX23X,RX72T
【选型】Renesas(瑞萨电子)MCU新产品型号速查手册(2018.3)
目录- 用于中国的MCU RL78系列MCU RX系列MCU
型号- R5F104PJGFA,R5F104PJGFB,R5F10JGCAFB,R5F104JHDLA,R5F101PJDFA,R7F0C206M2CFA-C,R5F564MLDDLC,R5F51303ADFK,R5F101FDAFP,R5F571MJGDLC,R5F104FDDFP,R5F564MLDDLK,R5F100LJAFA,R5F564MLDDLJ,R5F100BGGNA,R5F100LJAFB,R5F10RBAGFP,R5F571MFCDFP,R5F104GGGFB,R5F51303ADFP,R5F51303ADFM,R5F51303ADFL,R5F571MJGDLK,R5F51303ADFN,R5F571MJGDLJ,R5F100FCDFP,R5F5651EDDFC,R5F5651EDDFB,R5F571MFCDFB,R5F571MFCDFC,R5F5651EDDFP,R5F52305AGND,L13系列,R5F52305AGNE,R5F100GLDNA,R5F100EGANA,R5F51101AGFM,R5F100MLAFB,R5F565N7EGFP,R5F111NGALA,R5F100GFGFB,R5F100FDGFP,R5F100MLAFA,R5F101GFAFB,R5F104GFDFB,R5F564MFGDLC,R5F104BFAFP,R5F10EBCGNA,R5F10RFCAFP,R5F564MJDDBG,R5F101LLAFA,L1D系列,R5F101LLAFB,R5F100GADNA,R5F565N7EGFB,R5F100BFDNA,R5F110NEALA,R5F51101AGFK,R5F104GAANA,R5F10RF8AFP,R5F51101AGFL,R5F100AEGSP,R5F1056AGSP,R5F10266DSP,R5F10367ASP,R5F5651EDDLC,R5F100LJABG,R5F104ECGNA,R5F51103AGNE,R5F100GDAFB,R5F51103AGNF,R5F5651CDDBG,R5F10277ANA,R5F564MFGDLJ,R5F564MFGDLK,R5F5110JADNE,R5F101LEABG,R5F5110JADNF,G11,R5F104BGDFP,R5F104JGALA,R5F104FCAFP,R5F110NGGLA,R7F0C206L2DFAC,R5F1026ADSP,G1F,R5F100JFAFA,R7F00014L2DFA-C,R5F564MLHDBG,R5F117GAGFB,R5F56517FDFP,R5F100BEANA,R5F100ADDSP,R5F104CDALA,R5F56517FDFB,R7F0C014B2DFP-C,R5F111PFGFB,R5F100GKANA,R5F5651CDDFB,R5F564MJDDFP,R5F5110JADLF,R5F100CGALA,R7F0C902B2DFP-C,R5F101GGDNA,R5F104AADSP,R5F5651EDDLJ,R5F564MJDDFC,R5F564MJDDFB,R5F101SKAFB,R5F5110JADLM,R5F100GEDFB,R5F5651EDDLK,R5F100LKDFB,R5F104GEAFB,R5F100LKDFA,R5F10WLGAFA,R5F10WLGAFB,R5F11BGCAFB,R5F56519FDLK,R5F56519FDLJ,R5F571MLGDLK,R5F571MLGDLJ,R5F100LCABG,R7F0C206L2DFA-C,R5F10JGCANA,R5F100LDDFA,R5F100LDDFB,R5F104JCALA,R5F56517FDLK,R5F104FJAFP,R5F56517FDLJ,R5F100JJAFA,R5F5111JAGFM,R5F100ACASP,R5F102A8ASP,R5F101LEAFB,R5F101LEAFA,R5F100MGGFB,R5F100MGGFA,R5F52317BDND,R5F5111JAGFL,R5F52317BDNE,R5F5111JAGFK,R5F564MJDDLJ,R5F1006DDSP,R5F564MJDDLK,R5F571MLGDLC,R5F101MHDFB,R5F564MJDDLC,RX100,R5F101LFDFA,R5F100EADNA,R5F101LFDFB,R5F104AEDSP,R5F564MLHDFP,R5F100MFDFB,R5F100GFGNA,R5F100MFDFA,R5F101JLAFA,R5F101GFANA,R5F564MLHDFC,R5F565N4EDLJ,R5F565N4EDLK,R5F56514EGFB,R7F0C01072DNP,R5F564MLHDFB,R5F104GAAFB,R5F104LGAFP,R5F10WLCAFA,R5F56514EGFP,R5F10WLCAFB,R5F111MEAFB,R5F571MJGDFC,R5F571MJGDFB,R5F101BCANA,R5F104LGAFB,R5F104BFANA,R5F11B7CGNA,R5F104LGAFA,R5F101GKDFB,R5F100PGAFA,R5F100SJDFB,R5F565N9BGFB,R5F100PGAFB,R5F101CEALA,R5F571MJGDFP,R5F110MEGFB,R5F5110HADLM,R5F100GDANA,R5F51105AGNE,R5F100FGDFP,R5F104BAGFP,R5F10ELEGBG,R5F565N9BGFP,R5F104PFGFB,R5F101FHAFP,R5F564MLHDLJ,R5F564MLHDLK,R5F101BDDNA,R5F564MLHDLC,R5F51103AGFK,R5F571MJCDLK,R5F51103AGFL,R5F571MJCDLJ,R5F51103AGFM,R5F571MJCDLC,R7F0C01062DFP-C,R5F104AFGSP,R5F1017ADNA,R5F104BGDNA,R7F0C80112ESP,R5F104LGALA,R5F1007EANA,R5F104GCGNA,R5F11BGEGFB,R5F5651EDDBG,R5F571MFCDBG,R5F10JBCGFB,R5F52305AGFP,R5F565N7AGFP,R5F104EHANA,R5F104FEGFP,R5F10EGDAFB,R5F10WMEAFA,R5F5110HADNF,R5F52305AGFL,R5F52305AGFM,R5F52315CGND,R5F1006CASP,R5F101GJAFB,R5F52315CGNE,R5F10WMEAFB,R5F100FHGFP,R5F100GJGFB,R5F100PHDFB,R5F101MGAFB,R5F100PHDFA,R5F110PEAFB,R5F565N9ADFB,R5F100JKDFA,R5F571MJGDBG,R5F100GEDNA,R5F565N9ADFP,R5F100LEGFA,R5F564MJHDBG,R5F100LEGFB,R5F565N7AGFB,R5F10KBCAFB,R5F523T3ADFM,R5F100MJGFB,R5F523T3ADFL,R5F5651EHDLJ,R5F564MFDDBG,R5F5651EHDLC,R5F100MJGFA,R5F523T3ADFD,R5F100LFABG,R5F104EGGNA,R5F5651CHDBG,R5F101EEANA,R5F10RBCAFP,R5F571MLDDLC,R5F571MLDDLJ,R5F571MLDDLK,R5F104FGAFP,R5F101JEAFA,R5F104BEGFP,R5F111NFGLA,G1F系列,R5F10EBEANA,R5F10RB8AFP,R5F100AFASP,R5F571MJDDFB,R5F571MJDDFC,R5F51306AGFM,R5F51306AGFL,R5F51306AGFK,R5F101JFDFA,R5F100PJAFB,R5F100PJAFA,R5F1006ADSP,R5F52317BDFP,R5F571MJDDFP,R5F51306AGFP,R5F52317BDFL,R5F52317BDFM,R5F51306AGFN,R5F5651CHDFB,R5F5651CHDFC,R5F111PJGFB,R5F1027AGNA,R5F571MLHDBG,R5F100GGAFB,R5F564MJHDFP,R7F0C908B2DFP-C,R5F100EDDNA,R5F11BCCALA,R5F5651EHDLK,R5F10ELDGFB,R5F564MJHDFB,R5F104LDAFP,R5F564MJHDFC,R5F101EFDNA,R5F101GKDNA,R5F104EFDNA,R5F100LGDFB,R5F100LGDFA,R5F564MFDDFC,R5F564MFDDFB,R5F111MHGFB,R7F0C00BA2DSP,R5F104JFGLA,R5F100BCGNA,R5F564MFDDFP,R5F5651CHDFP,R5F1008DALA,R5F110PGGFB,R5F52316AGNE,R5F52316AGND,R5F565N9EGFB,RX113,R7F0C901B2DNP-C,R5F10EGCANA,R5F104BAGNA,RX110,RX111,R5F100FJDFP,R5F111PHAFB,R7F0C905B2DFP-C,R5F51114ADNE,R5F565N9EGFP,R5F100PKDFB,R7F0C008A2DSP,R5F100PKDFA,R5F564MJHDLK,R5F104MFAFB,R5F51105AGFM,R5F564MJHDLJ,R5F104MFAFA,R5F101MLDFB,R5F564MJHDLC,R5F51105AGFK,R5F104LJGFP,R5F51105AGFL,R5F104GDGFB,R5F101GDDFB,R5F10WLFGFB,R5F565N4BDLK,R5F571MJDDBG,R7F0C80862ESN-C,R5F565N4BDLJ,R5F100GHDNA,R5F571MFGDLC,R5F101LJDFB,R5F104LJGFA,R5F100ECANA,R5F101LJDFA,R5F104LJGFB,R5F100LHGFA,R5F100JCDFA,R5F100LHGFB,R5F104GJDNA,R5F104LDALA,R5F101GJANA,R5F104EEANA,R5F52315CGFL,R5F52315CGFM,RX130,R5F52315CGFP,R5F10KBCANA,R5F104ADASP,R5F110MJAFB,R5F52317BDLA,R5F51114ADLF,R5F571MFGDLJ,R5F571MFGDLK,R5F101CAALA,R7F0C002G2DFB,R5F100AAGSP,R5F10269ASP,R5F101FAAFP,R5F565N4BDFB,R5F102A7GSP,R5F10RLCGFB,R5F5111ADNF,R5F10RLCGFA,R5F5651CDDFC,R5F101FLAFP,R5F5651CDDFP,R5F51303ADNE,R5F101GCAFB,R5F10368DSP,R5F104BEGNA,R5F1017CANA,R5F5651EHDBG,R5F571MFGDBG,R5F10379ANA,L1X系列,R5F104LFGFP,R5F11BCEGLA,R5F104GGGNA,R5F101PFAFA,R5F564MFDDLC,R5F110NHALA,R5F564MFDDL
【IC】瑞萨推出全新超高性能产品业界首款基于Arm® Cortex®-M85处理器的MCU RA8系列
瑞萨RA8系列MCU是业界首款采用Arm® Cortex®-M85处理器的产品,提供卓越6.39 CoreMark/MHz(注)性能——这一性能水平将使系统设计人员能够使用RA MCU替代应用中常用微处理器。
产品 发布时间 : 2023-11-02
【经验】解析瑞萨MPU RZ/T1 SPI总线通信功能配置方法
当我们使用瑞萨(Renesas)MPU RZ/T1做项目开发时,基本都会用到SPI总线通信的功能,SPI总线通信速率较快很适合在单片机板级之间进行数据交互,本文将介绍使用瑞萨开发环境配置生成RZ/T1 SPI总线驱动程序的方法。
设计经验 发布时间 : 2022-05-02
【经验】瑞萨MPU RZ/T1使用SSC生成EtherCAT CiA402协议栈方法
瑞萨MPU RZ/T1支持EtherCAT网络设计,如何使用协议栈工具生成相对应的EtherCAT从机栈代码呢,本文主要就Beckhoff工具生成EtherCAT CiA402协议栈方法做相关介绍。
设计经验 发布时间 : 2021-12-26
瑞萨RX系列MCU实现二级MCU OTA升级:FreeRTOS篇
基于空中下载技术(OTA:Over-The-Air)并通过云服务端实现的MCU固件升级得益于云供应商和设备供应商之间的合作开展,目前已具备了简单且可快速部署的集成环境。瑞萨RX云互联解决方案开发团队开始提供即便在未直接与互联网连接的二级MCU中,也可以利用AWS IoT服务方案进行OTA固件升级的示例代码。
原厂动态 发布时间 : 2022-05-24
【经验】瑞萨RZ/T2M系列MPU使用IAR开发环境时的调试方法和注意事项
瑞萨RZ/T2M系列MPU可以使用IAR开发环境进行开发,当我们使用IAR开发环境加载一些瑞萨RZ/T2M相关例程项目时,总是会遇到一些编译报错问题,烧写异常问题,此文主要介绍使用IAR开发环境连接调试RZ/T2M系列MPU的方法和一些注意事项。
设计经验 发布时间 : 2023-03-31
RZ/G MPU G2L,LC,UL GROUP
型号- RZ/G3YYY,RZ/G2L,RZ/G2M,RZ/G2LC,RZ/G2UL,RZ/G1M,RZ/G2N,RZ/G1N,RZ/G,RZ/G1C,RZ/G2E,RZ/G1E,RZ/G2H,RZ/G1H,RZ/G3YY,RZ/G3XX,RZ/G3ZZ,RZ/G4X,RZ/G3X,RZ/G3Y,RZ/G4XX,RZ/G3Z
【经验】瑞萨MPU RZ/T1使用code generator生成SCI的初始代码方法
工程师初次使用瑞萨(Renesas)RZ/T1系列MPU时,可能不知道SCI该如何配置波特率,中断入口,中断标志位等。本文将介绍RZ/T1系列MPU利用code generator生产SCI的配置方法。
设计经验 发布时间 : 2022-04-22
【选型】Renesas(瑞萨电子) RL78系列16位MCU选型指南
目录- RL78家族特点 通用型MCU LCD MCU ASSP 智能模拟 汽车 开发环境&开发工具 解决方案 产品一览表 封装
型号- R5F104PFAFA,R5F101PHAFB,R5F104PFAFB,R5F10BAFLSP,R5F101PHAFA,R5F113TJLFB,R5F110MEAFB,R5F10JGCAFB,R5F10AACLSP,R5F10AGCLNA,R5F111MHAFB,R5F10WMGAFA,R5F10WMGAFB,R5F101ACASP,R5F104JHAFA,R5F101JJAFA,R5F104BDANA,R5F101BFANA,R5F10PADLSP,R5F1006EASP,R5F101FDAFP,R5F100LJAFA,R5F100LJAFB,R5F100JGAFA,R5F101LCABG,R5F104CFALA,R5F1018CALA,R5F100BCANA,R5F10Y14ASP,R5F100FLAFP,RL78-S2,R5F100EHANA,R5F11MMDAFB,R5F10PGFLFB,R5F100MKAFB,R5F100MKAFA,R5F100CEALA,R5F10BLELFB,R5F100FAAFP,R5F104AAASP,R5F100SJAFB,RL78/F1X,R5F113GLLFB,R5F10BGFLNA,R5F10WLEAFB,R5F104GCAFB,R5F10KGCANA,R5F10WLEAFA,R5F101GCANA,R5F110PJAFB,R5F11BGEAFB,R5F10369ASP,R5F10BMFLFB,R5F100EGANA,R5F113MKLFB,R5F104LJAFP,R5F100GJANA,R5F10278ANA,R5F100MLAFB,R5F1176AGSP,R5F11FLLANA,R5F100FKAFP,R5F100CFALA,R5F10AGELFB,R5F111NGALA,R5F104LJAFA,R5F104LJAFB,R5F10JBCANA,R5F104LHALA,R5F100MLAFA,R5F101SLAFB,R5F101GFAFB,R5F10ALCLFB,R5F10WLFAFB,R5F104BFAFP,R5F104GDAFB,R5F10WLFAFA,R5F11EF8AFP,R5F10PPHLFB,R5F104GLANA,R5F10BGGLNA,R5F1017AANA,R5F10AADLSP,R5F10RFCAFP,R5F110MFAFB,R5F101LLAFA,R5F104JGAFA,R5F104BCANA,R5F1006DASP,R5F10NLE/G,R5F1008AALA,R5F101FEAFP,R5F110NEALA,R5F100AAASP,R5F104GAANA,R5F10RF8AFP,R5F11EAAASP,R5F100JHAFA,R5F10MPEDFB,R5F100BDANA,R5F104CEALA,R5F104MKAFA,R5F10MPGDFB,R5F104MKAFB,R5F10PGELFB,R5F113GKLNA,R5F11MMEAFB,R5F100GLANA,R5F10367ASP,R5F100MJAFA,R5F100MJAFB,R5F10AGGLFB,R5F117BAGFP,R5F10NPJDFB,R5F100LJABG,R5F100GDAFB,R5F104LLAFB,R5F104LLAFA,R5F104LJALA,R5F100SKAFB,R5F101GDANA,R5F10PPJLFB,R5F101PGAFA,R5F10277ANA,R5F10BAELSP,R5F113TKLFB,R5F101PGAFB,R5F101LEABG,R5F10AGDLNA,R5F100GAANA,R5F10NMJ/G,R5F107AEMSP,RL78/G10,R5F101BEANA,RL78/G11,R5F10PMFLFB,RL78/G12,RL78/G13,RL78/G14,R5F101GGAFB,R5F104BEAFP,R5F104FCAFP,R5F101CGALA,R5F100JFAFA,R5F113TLLFB,R5F101LDABG,R5F10BADLSP,R5F11BLCAFB,RL78/G1A,RL78/G1C,RL78/G1D,RL78/G1E,RL78/G1F,RL78/G1G,RL78/G1H,R5F117GAGFB,R5F10BLFLFB,R5F101AAASP,R5F10PMELFB,R5F101BDANA,R5F10RLAAFB,R5F101FFAFP,R5F10AAELSP,R5F10EGAAFB,R5F100BEANA,R5F101CFALA,R5F104FDAFP,R5F10RLAAFA,R5F10Y16ASP,R5F104CDALA,R5F10368ASP,R5F10PGDLFB,R5F104MLAFB,R5F10BMGLFB,R5F11MMFAFB,R5F100GKANA,R5F104MLAFA,R5F101JKAFA,R5F101LCAFA,R5F11768GSP,R5F101LCAFB,R5F100CGALA,R5F10AGFLFB,R5F111NHALA,R5F101GEANA,R5F110PHAFB,R5F100GCAFB,R5F104LKAFB,RL78/F12,RL78/F13,R5F101SKAFB,R5F11B7CANA,R5F11CBCGNA,RL78/F14,R5F104LKAFA,RL78/F15,R5F100SLAFB,R5F1018DALA,R5F104GEAFB,RL78/G1X,R5F10WLGAFA,R5F10WLGAFB,R5F11BGCAFB,R5F101EHANA,R5F101LFABG,R5F104LHAFP,R5F10FMCAFB,R5F10Y46ASP,R5F11BLEAFB,R5F100LCABG,R5F104BGANA,R5F10AGCLFB,R5F10JGCANA,R5F104JEAFA,R5F110PGAFB,R5F104LHAFB,R5F104LHAFA,R5F100PHAFA,R5F100PHAFB,R5F101GHAFB,R5F104LFALA,R5F10PPFLFB,R5F10268ASP,R5F113TGLFB,R5F10BGCLNA,R5F104FJAFP,R5F100JJAFA,R5F113GLLNA,R5F104MJAFA,R5F100ACASP,R5F104MJAFB,R5F10ABDLNA,R5F102A8ASP,R5F1016EASP,R5F101LEAFB,R5F101LEAFA,R5F101PKAFA,R5F101PKAFB,R5F100FDAFP,R5F111NEALA,R5F10PGFLNA,R5F101FGAFP,R5F100GEAFB,R5F10BGFLFB,R5F101MFAFA,R5F104EFANA,R5F101MFAFB,R5F11MPFAFB,R5F100BFANA,R5F104GKAFB,R5F10E8DALA,R5F10AAALSP,RL78/I1A,RL78/I1B,RL78/I1C,RL78/I1D,R5F101LDAFA,RL78/I1E,R5F104ACASP,R5F101LDAFB,R5F101JLAFA,R5F101GFANA,R5F101EGANA,R5F11MPEAFB,R5F104GLAFB,R5F104GAAFB,R5F104LGAFP,R5F10RBAAFP,R5F10WLCAFA,R5F10WLCAFB,R5F111MEAFB,R5F10PGHLFB,R5F113LLLFB,R5F101BCANA,R5F104LGAFB,R5F107ACMSP,R5F104BFANA,R5F10WMDAFB,R5F11CBCMNA,R5F100FCAFP,R5F104LGAFA,R5F10WMDAFA,R5F100PGAFA,R5F100SHAFB,R5F104LEALA,R5F100PGAFB,R5F10PPELFB,R5F101CEALA,R5F10BGDLNA,R5F113THLFB,RL78/I1X,R5F100ADASP,R5F10ABELNA,R5F10WLDAFB,RL78/L1A,R5F100GDANA,R5F11FLJANA,R5F101LFAFB,RL78/L1C,R5F100FEAFP,R5F100EAANA,R5F101LFAFA,R5F10PGELNA,R5F101FHAFP,R5F10BGGLFB,R5F117BAGNA,R5F101CDALA,R5F11MPGAFB,R5F10RLCAFA,R5F104EGANA,R5F10RLCAFB,R5F10WLDAFA,R5F100BGANA,R5F11EFAAFP,R5F100LKAFA,R5F11EA8ASP,R5F111MGAFB,R5F104GJANA,R5F10AGDLFB,R5F10EGCAFB,R5F10WMFAFA,R5F10WMFAFB,RL78/L1E,R5F10MMEDFB,R5F10BLDLFB,R5F111NFALA,R5F101GGANA,R5F11BCEALA,R5F104LGALA,R5F1007EANA,R5F10267ASP,R5F100LKAFB,R5F110PFAFB,R5F104CGALA,R5F10BMELFB,R5F10PPGLFB,RL78/L1X,R5F101EFANA,R5F10PAELSP,R5F100LLAFB,R5F10AGALNA,R5F10PGGLFB,R5F111MFAFB,R5F100LLAFA,R5F104EHANA,R5F104GKANA,R5F102A9ASP,R5F113LKLFB,RY7011A0000DZ00,R5F10EGDAFB,R5F101PJAFA,R5F101PJAFB,R5F10A6ALSP,R5F10KBCAFP,R5F10WMEAFA,R5F104JFAFA,R5F10BLCLFB,R5F117BCGFP,R5F104BEANA,R5F1006CASP,R5F100GFAFB,R5F101GJAFB,R5F10WMEAFB,R5F100PFAFB,R5F101MGAFB,R5F10BGELNA,R5F100PFAFA,R5F10266ASP,R5F10RLAANB,R5F110PEAFB,R5F1018AALA,R5F101MGAFA,R5F100AEASP,R5F10EGAANA,R5F101LGABG,R5F10E8EALA,R5F10FMDAFB,R5F10Y47ASP,R5F104BGAFP,R5F10279ANA,R5F10BAGLSP,R5F113MLLFB,R5F10PGDLNA,R5F100GCANA,R5F111PJAFB,R5F11FLKANA,R5F11BBEAFP,R5F101JCAFA,R5F1026AASP,R5F10RJAAFA,R5F104FAAFP,R5F10NMJDFB,R5F107DEMSP,R5F110NJALA,R5F100MFAFB,R5F100MFAFA,R5F1037AANA,R5F1007CANA,R5F111PGAFB,R5F10A6CLSP,R5F10PMJLFB,R5F100GHAFB,R5F10BBDLNA,R5F10BGCLFB,R5F104GFANA,R5F100LFABG,R5F104MGAFB,R5F104MGAFA,R5F101GHANA,R5F104ECANA,R5F104LEAFP,R5F101EEANA,R5F10RBCAFP,R5F104AFASP,R5F104PKAFB,R5F101BAANA,R5F100GEANA,R5F104LEAFA,RL78/G1,R5F104LEAFB,R5F104FGAFP,R5F101GKAFB,R5F101JEAFA,R5F11BBCAFP,R5
电子商城
现货市场
服务
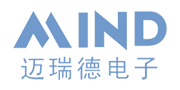
可定制显示屏的尺寸0.96”~15.6”,分辨率80*160~3840*2160,TN/IPS视角,支持RGB、MCU、SPI、MIPI、LVDS、HDMI接口,配套定制玻璃、背光、FPCA/PCBA。
最小起订量: 1000 提交需求>
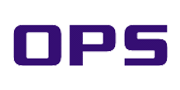
可烧录IC封装SOP/MSOP/SSOP/TSOP/TSSOP/PLCC/QFP/QFN/MLP/MLF/BGA/CSP/SOT/DFN;IC包装Tray/Tube/Tape;IC厂商不限,交期1-3天。支持IC测试(FT/SLT),管装、托盘装、卷带装包装转换,IC打印标记加工。
最小起订量: 1pcs 提交需求>
登录 | 立即注册
提交评论